Featured news
See all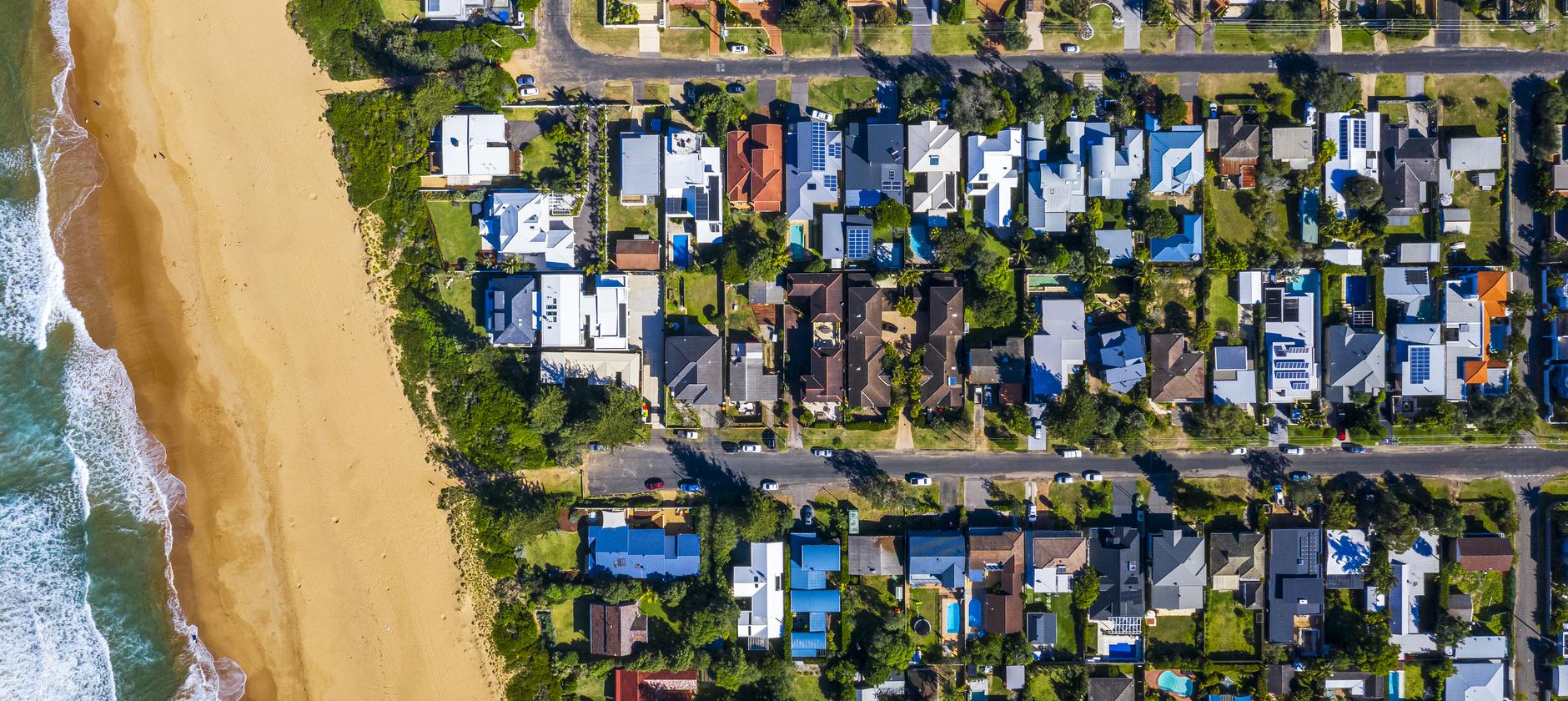
Article
Auction Market Preview; Research News
Auction Market Preview - 6 July 2025
Winter school holidays chills auction activity
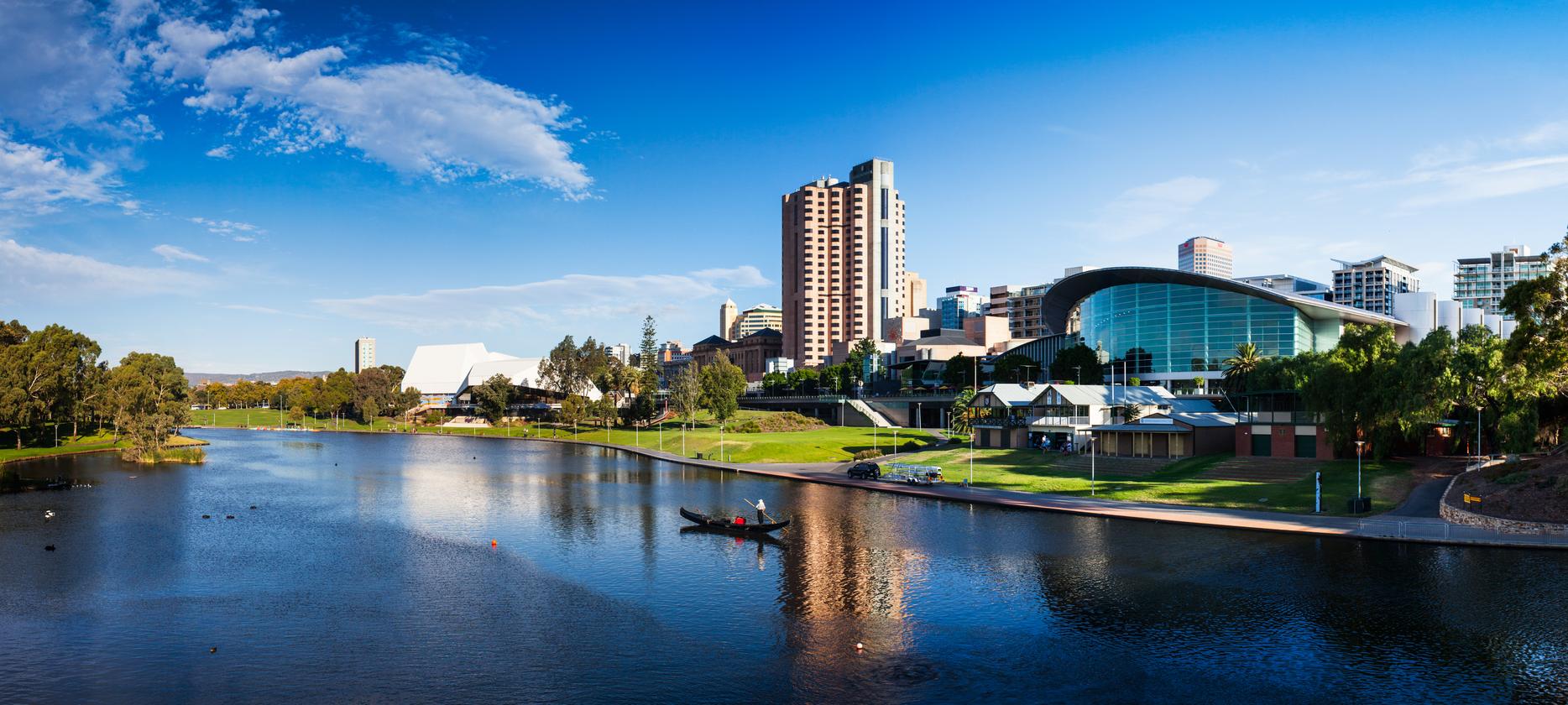
Article
Auction Market Preview, Research News
Auction Market Preview - 29 June 2025 Auction Market Preview - 29 June 2025
Reports
See all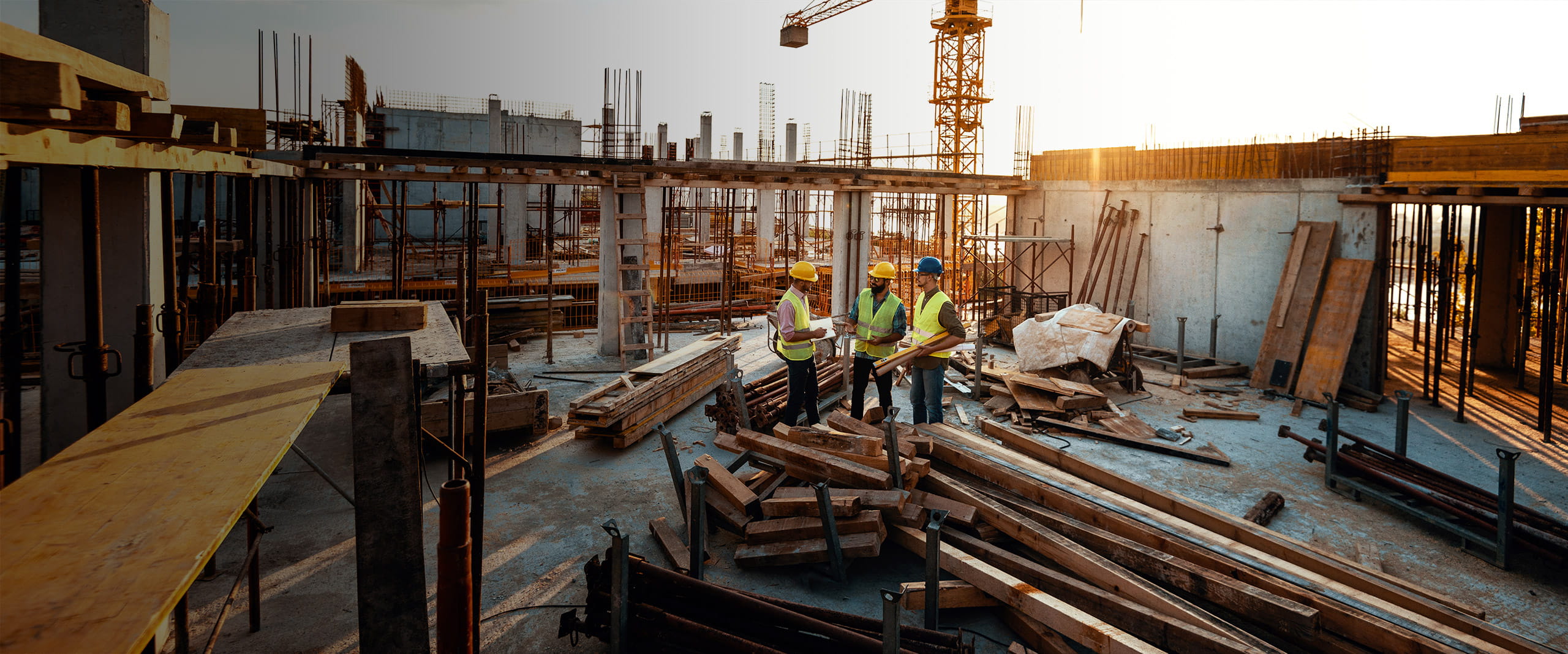
Report
Commercial Pulse
Cordell Construction Monthly Report
Cordell Construction Monthly provides a regular update on the number and value of construction projects that are in planning or have commenced construction across residential, community, commercial and major infrastructure developments.
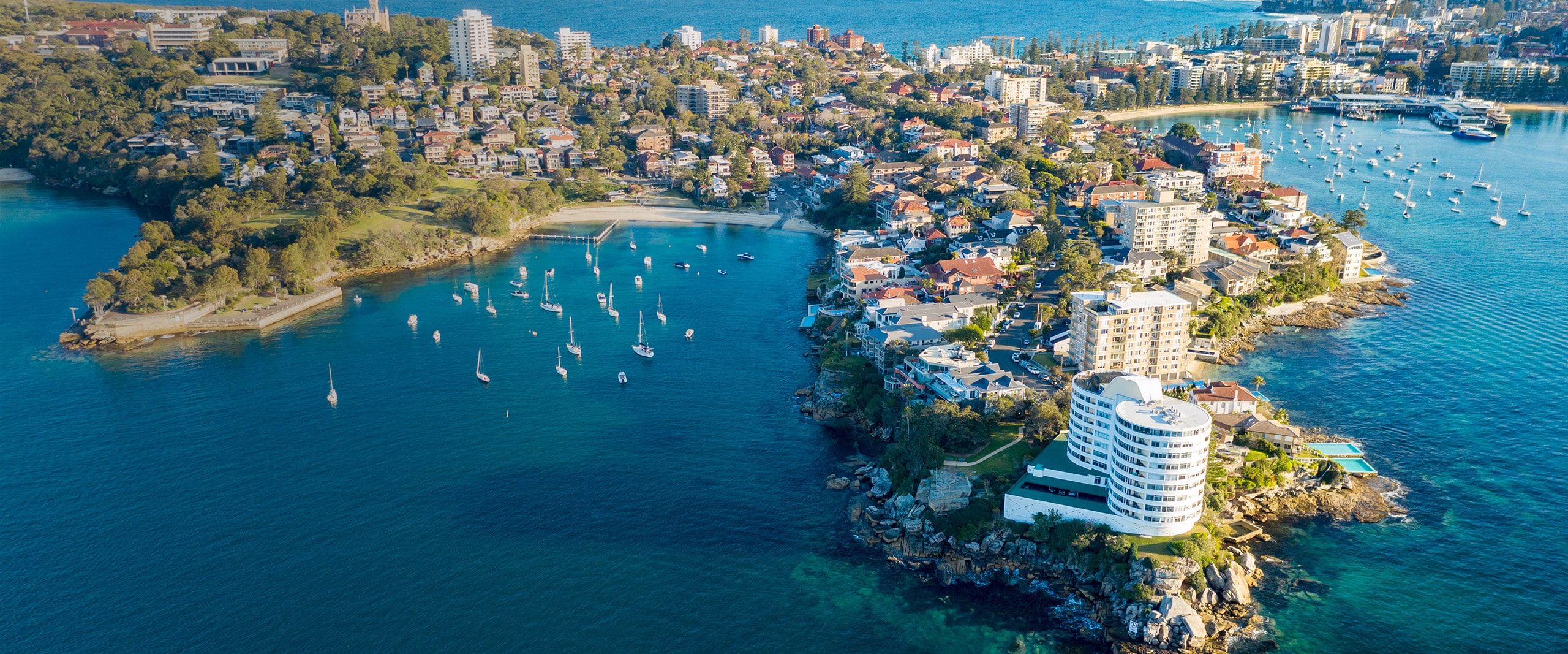
Report
Thought Leadership
Pain & Gain Report Pain & Gain Report
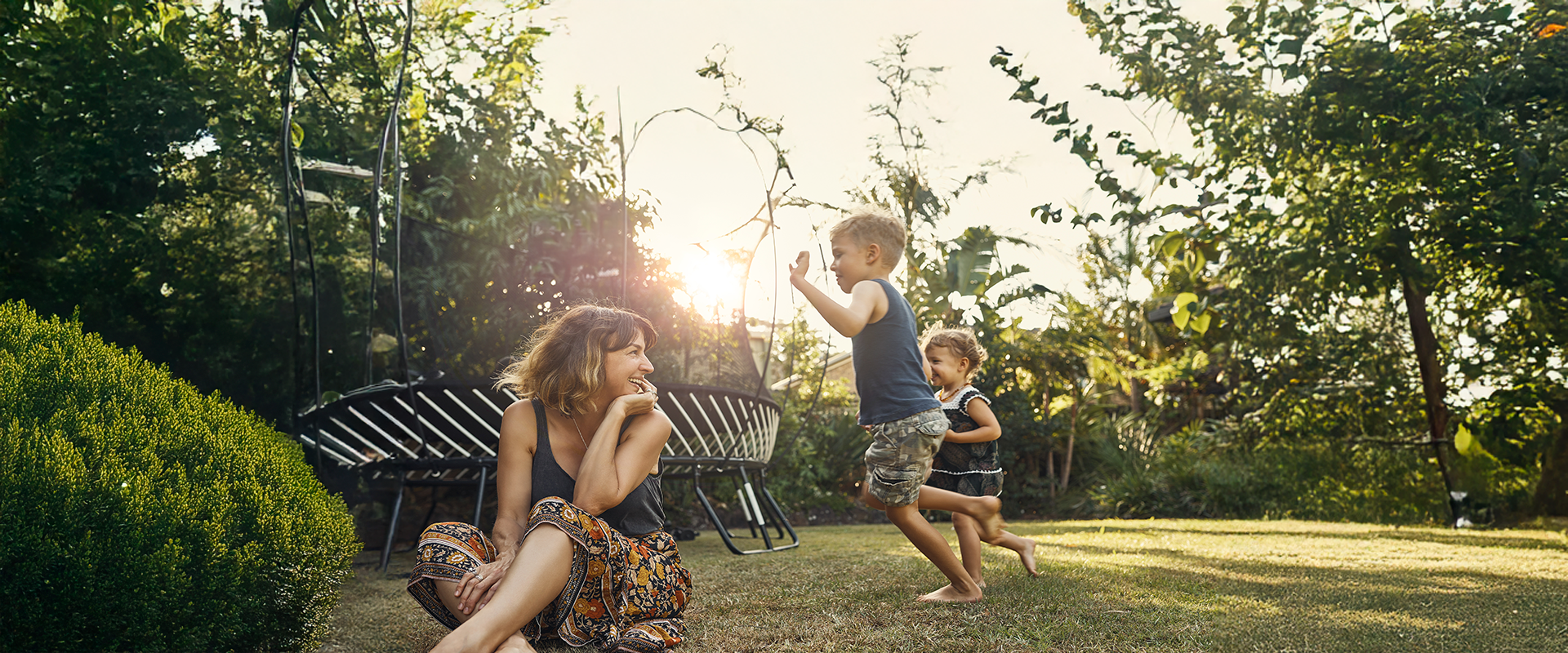
Report
Research News, Thought Leadership, Women and Property
Women and Property 2025 Women and Property 2025
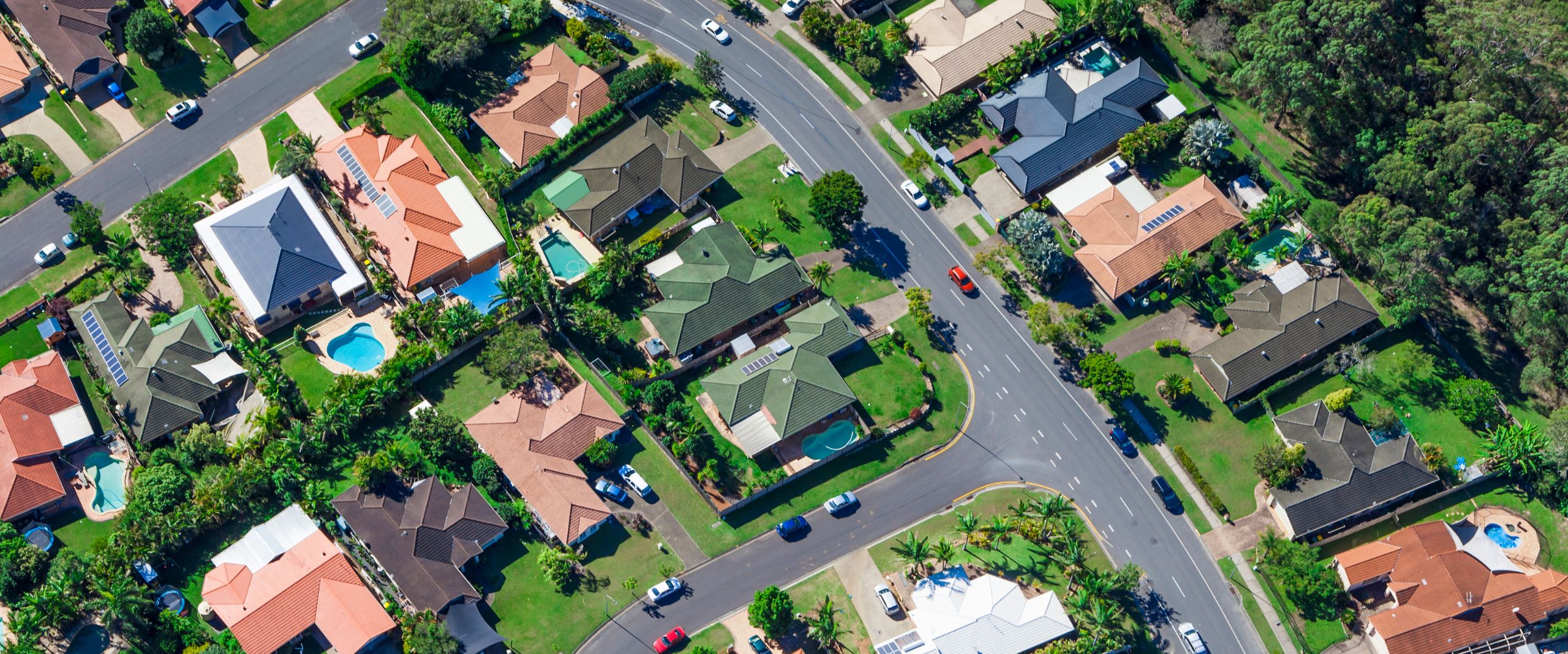
Report
Product News, Property Pulse, Thought Leadership
Data-driven insights and strategies from real estate age… Data-driven insights and strategies from real estate agents
Product News
See all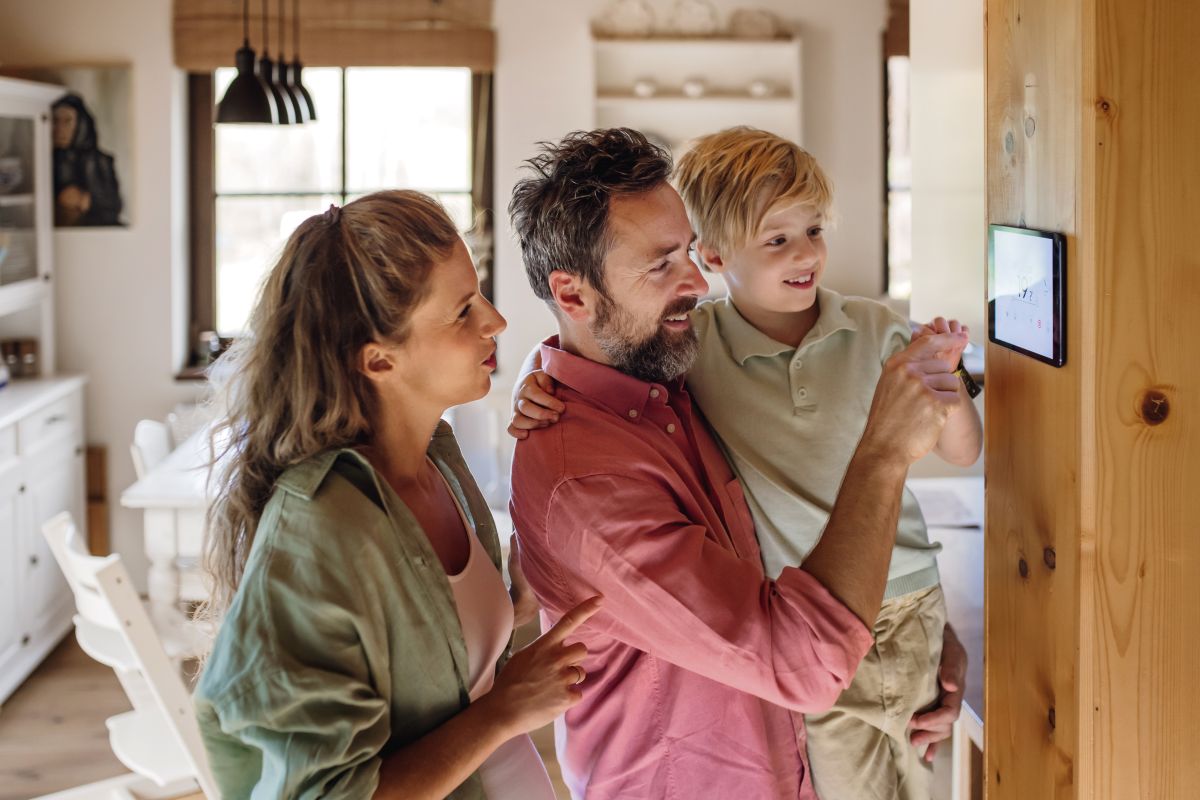
Article
Product News, Property Resilience